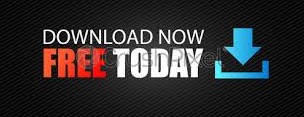
- Docear user manual how to#
- Docear user manual pdf#
- Docear user manual software#
- Docear user manual Offline#
- Docear user manual free#
To create recommendations, the recommender system randomly chooses one of three recommendation approaches. In addition, users can request recommendations at any time. Docear users, who agree to receive recommendations, automatically receive recommendations every five days upon starting the program. Clicking the circle would unfold the node, i.e. A circle at the end of a node indicates that the node has child nodes, which are hidden ("folded").
Docear user manual pdf#
title and journal name), , and displays metadata when the mouse hovers over a PDF icon. Docear also extracts metadata from PDF files (e.g. Docear import ed annotations (comments, highlighted text, and bookmarks) made in the PDFs, and clicking a PDF icon opens the linked PDF file. We created categories reflecting our research interests (“Academic Search Engines”), sub- categories (“Google Scholar”), and sorted PDFs by category and subcategory.
Docear user manual how to#
Figure 1 shows an example mind-map that shows how to manage PDFs and references in Docear. Clicking a recommendation opens the paper in the user’s web browser. Recommendations are displayed as a list of ten research papers, showing the title of the recommended papers. Since 2012, Docear has been offering a recommender system for 1.8 million publically available research papers on the web. It has approximately 20,000 registered users and uses mind-maps to manage PDFs and references.
Docear user manual free#
Docear is a free and open-source literature suite, used to organize references and PDFs.
Docear user manual Offline#
We evaluated the effectiveness of the approaches and variations with an offline evaluation, online evaluation, and user study, and compared the results.
Docear user manual software#
To achieve the research objective, we implemented different recommendation approaches and variations in the recommender system of our literature management software Docear. Compared to our previous paper, the current paper is more comprehensive, covers three instead of two evaluation methods, considers more metrics, is based on more data, and provides a deeper discussion. In addition, we are first to discuss the appropriateness of the evaluation methods in the context of research-paper recommender systems, aside from our previous paper on recommender system evaluation. To the best of our knowledge, we are first to compare the results of all three evaluation methods, and to discuss the adequacy of the methods and metrics in detail. Our research goal hence was to explore the adequacy of online evaluations, user studies, and offline evaluations. In addition, the existing comparisons in other recommender disci- plines focus on offline evaluations and user studies, , or offline evaluations and online evaluations, but not on all three methods. In the field of research-paper recommender systems, there is no research or discussion about how to evaluate recommender systems. students) to participate in a user study. In addition, both user studies and online evaluations require significantly more time than offline evaluations, and can only be conducted by researchers who have access to a recommender system and real users, or at least some participants (e.g. showed that CTR and relevance do not always correlate and concluded that "CTR may not be the optimal metric for online evaluation of recommender systems" and "CTR should be used with precaution". For instance, results of user studies may vary, depending on the questions. However, online evaluations and user studies are also not without criticism. This is also true in the field of research-paper recommender systems, where the majority of recommendation approaches are evaluated offline, and only 34% of the approaches are evaluated with user studies and only 7% with online evaluations. Despite the criticism, offline evaluations are the predominant evaluation method in the recommender community. For instance, users may be dissatisfied with recommender systems, if they must wait for too long to receive recommendations, or the presentation is unappealing. The main reason for the criticism in the literature is that offline evaluations ignore human factors, yet human factors strongly affect overall user satisfaction with recommendations. Others believe that "online evaluation is the only technique able to measure the true user satisfaction". reported that "the presumed link between algorithm accuracy and user experience is all but evident". of o ffl ine may remain inconclusive or even misleading" and "real-world evaluations and, to some extent, lab studies represent probably the best methods to evaluate systems".
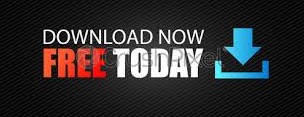